Línea de Investigación
Procesamiento de Señales, Imágenes e Inteligencia Artificial
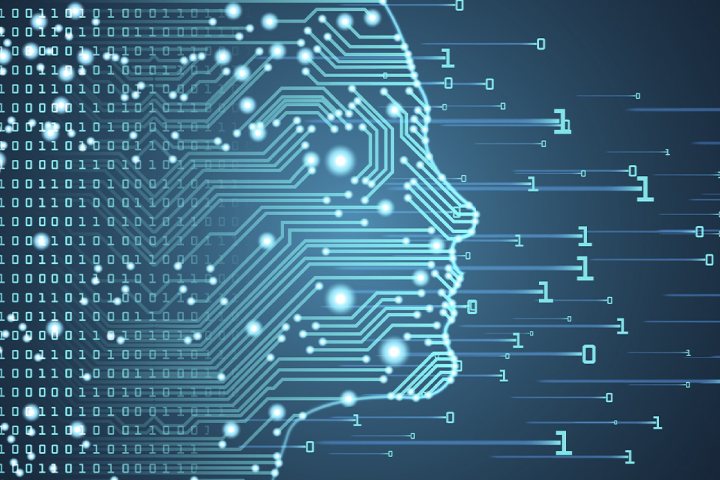
Trayectoria:
El grupo ha surgido en base a los investigadores de la Coordinación II, I+D+i, de la Dirección de Investigación y Desarrollo Tecnológico DIDT, de la Unidad Ejecutora 002 – INICTEL-UNI. El grupo se ha ido consolidando a lo largo de los últimos años con trabajos multidisciplinarios e interinstitucionales a través de convenios con el Instituto Nacional de Investigación Agraria, Instituto de Investigación de la Amazonia Peruana, Universidad Castilla de la Mancha y el Programa Conservación del MINAM.
Objetivos:
- Generar y/o aplicar conocimiento en TIC para contribuir con la resolución de problemas en bien de la sociedad.
- Desarrollar y difundir soluciones de hardware y software para apoyar a productores y especialistas de instituciones públicas o privadas en la toma de decisiones.
Líneas de Investigación:
Las áreas de conocimiento más estudiadas por el grupo son procesamiento de señales e imágenes, inteligencia computacional, estadística y/o sistemas de información concentradas en las siguientes líneas de investigación:
- Teledetección: procesamiento de imágenes satelitales y de UAV, sistemas de información georreferenciada, sistemas informáticos para agricultura y recurso naturales, procesamiento de señales e imágenes de radares y acústicas, sistemas de comunicaciones y prototipos para la gestión de riego de desastres.
- Biomédica: procesamiento de imágenes médicas, procesamiento de señales bioeléctricas, reconocimiento e identificación de rostros.
- Inteligencia artificial: Inteligencia computacional, específicamente, redes neuronales artificiales, aprendizaje profundo, lenguaje natural, computación evolucionaria.
Servicios Ofrecidos y Realizados:
- Adquisición y procesamiento de imágenes aéreas con la finalidad de determinar el estado de cultivos
- Capacitación en agricultura de precisión usando drones y TIC
- Reconstrucción 3D de zonas arqueológicasy de cuenca hidrográficas
- Desarrollo de software para procesamiento de imágenes satelitales para el área forestal.
Correo de contacto
[email protected]Equipo
-
Joel Enyelber Telles Castillo
Procesamiento Digital de Imágenes, Teledetección y Compresión de Imágenes
Proyectos
Publicaciones:
2019
- Arteaga, D., Kemper, G., Huamán, S., Telles, J., Bendayán, L. and Sanjurjo, J.: A Method for Mosaicking Aerial Images based on Flight Trajectory and the Calculation of Symmetric Transfer Error per Inlier. In: Advances in Science, Technology and Engineering Systems Journal (ASTESJ): Special Issue on Multidisciplinary Sciences and Engineering. DOI: 10.25046/aj040642
- Salazar-Reque, I. F. and Huaman-Bustamante, S. G. (accepted): Automatic Leaf Segmentation from Images Taken Under Uncontrolled Conditions Using Convolutional Neural Networks. In: 5th Brazilian Technology Symposium – BTSym2019.
- Lopez, L., Apolinario, M. and Huaman-Bustamante, S. G. (accepted): Method of estimating river levels using artificial vision techniques and reflective tapes as a reference. In: 5th Brazilian Technology Symposium – BTSym2019.
- Rivadeneyra, B. and Huaman-Bustamante, S. G. (accepted): Method of Anomalies Detection in Persea Americana Leaves with Thermal and NGRDI Imagery. In: 5th Brazilian Technology Symposium – BTSym2019.
- Apolinario, M., Urcia, D. and Huaman-Bustamante, S. G.: Open Set Recognition of Timber Species Using Deep Learning for Embedded Systems. In: IEEE Latin America Transactions: Special Issue on Deep Learning. DOI: 10.1109/TLA.2019.9011545
- Huamán-Bustamante, S.G., Castro A., Morales G. and Telles J.: Regression Models between Active Sensor-Measured NDVI and UAV-Acquired Multispectral Images with Positioning Uncertainty. In: IEEE Latin America Transactions. DOI: 10.1109/TLA.2019.8896829
- Kemper, G., Ponce, D., Telles, J. and Del Carpio, C..: An Algorithm to Obtain Boat Engine RPM from Passive Sonar Signals Based on DEMON Processing and Wavelets Packets Transform. In: Journal of Electrical Engineering & Technology (2019). https://doi.org/10.1007/s42835-019-00260-4
- A. Anghel, M. Tudose, R. Cacoveanu, M. Datcu, G. Nico, O. Masci, A. Dongyang, W. Tian, C. Hu, Z. Ding, H. Nies, O. Loffeld, D. Atencia, S. G. Huaman, A. Medella, J. Moreira.: Compact Ground-Based Interferometric Synthetic Aperture Radar: Short-range structural monitoring. In: IEEE Signal Processing Magazine, vol. 36, no. 4, pp. 42-52, July 2019. DOI: 10.1109/MSP.2019.2894987
- Apolinario, M., Huaman-Bustamante, S., Morales, G. and Díaz, D.: Estimation of 2D Velocity Model using Acoustic Signals and Convolutional Neural Networks. In: IEEE XXVI International Conference on Electronics, Electrical Engineering and Computing (INTERCON), 2019. DOI: 10.1109/INTERCON.2019.8853566
- Morales, G., Ramirez, A. and Telles, J.: End-to-end Cloud Segmentation in High-Resolution Multispectral Satellite Imagery Using Deep Learning. In: IEEE XXVI International Conference on Electronics, Electrical Engineering and Computing (INTERCON), 2019. DOI: 10.1109/INTERCON.2019.8853549
- Morales G., Salazar-Reque I., Telles J., Díaz D.: Detecting Violent Robberies in CCTV Videos Using Deep Learning. In: MacIntyre J., Maglogiannis I., Iliadis L., Pimenidis E. (eds) Artificial Intelligence Applications and Innovations. AIAI 2019. IFIP Advances in Information and Communication Technology, vol 559. Springer, Cham.
- Vargas-Florez J., Palomino G. Flores A., Valdivia G., Saito C., Arteaga D., Balcazar M., Fernandez M., Oliden J., Díaz D.: Identifying potential landslide location using Unmanned Aerial Vehicles (UAVs). In:16th International Conference on Information Systems for Crisis Response and Management (ISCRAM 2019). ISBN 978-84-09-10498-7 / ISSN 2411-338
- Ramirez, A., Valle, G., Valbuena, L.: Seismicity risks by water injection induced for extraction of crude oil. In: XXII Symposium on Image, Signal Processing and Artificial Vision (STSIVA 2019).
- Ramírez A., Pacheco, A., Telles J.: Mapping vegetation, water bodies and urban areas in PeruSAT-1 satellite imagery. In: XXII Symposium on Image, Signal Processing and Artificial Vision (STSIVA 2019).
- Salazar-Reque, I. F., Pacheco, A.G., Rodriguez, R. Y., Lezama, J., and Huaman-Bustamante, S.: An image processing method to automatically identify Avocado leaf state. In: XXII Symposium on Image, Signal Processing and Artificial Vision (STSIVA 2019).
- Salazar-Reque, I. F., Kemper, G., Huaman-Bustamante, S. G., Telles, J., and Diaz, D.: An Algorithm for Plant Disease Visual Symptom Detection in Digital Images based on Superpixels. International Journal on Advanced Science, Engineering and Information Technology, 9(1), pp. 194-203, DOI:10.18517/ijaseit.9.1.5322
2018
- Morales, G., Kemper G., Sevillano G., Arteaga D., Ortega I., and Telles J.: Automatic Segmentation of Mauritia flexuosa in Unmanned Aerial Vehicle (UAV) Imagery Using Deep Learning. Forests. 9 (12), 736 (2018). DOI: https://doi.org/10.3390/f9120736 (Q1 journal)
- Huamán, S.G., Cavalcanti, M.A. and Lazo, J.G.: Estimation of the displacements among distant events based on parallel tracking of events in seismic traces under uncertainty. Computers & Geosciences, vol. 116. pp. 81 – 90, 2018. (Q1 journal)
- Morales G., Huamán S.G. and Telles J.: Shadow Removal in High-Resolution Satellite Images Using Conditional Generative Adversarial Networks. In: Lossio-Ventura J., Muñante D., Alatrista-Salas H. (eds) Information Management and Big Data. SIMBig 2018. Communications in Computer and Information Science, vol 898. Springer, Cham. DOI: 10.1007/978-3-030-11680-4_31
- Apolinario M.P., Huamán S.G., Clostre G.: Deep Learning Applied to Identification of Commercial Timber Species from Peru. In: IEEE XXV International Conference on Electronics, Electrical Engineering and Computing (INTERCON), 2018. DOI: 10.1109/INTERCON.2018.8526457
- Palomino, W., Morales, G., Huamán, S. and Telles, J.: PETEFA: Geographic Information System for Precision Agriculture. In: IEEE XXV International Conference on Electronics, Electrical Engineering and Computing (INTERCON), 2018. DOI: 10.1109/INTERCON.2018.8526414
- Morales, G., Arteaga, D., Huamán, S., Telles, J. and Palomino, W.: Shadow Detection in High-Resolution Multispectral Satellite Imagery Using Generative Adversarial Networks. In: IEEE XXV International Conference on Electronics, Electrical Engineering and Computing (INTERCON), 2018. DOI: 10.1109/INTERCON.2018.8526416
- Morales G., Huamán S.G. and Telles J.: Cloud Detection in High-Resolution Multispectral Satellite Imagery Using Deep Learning. In: Kůrková V., Manolopoulos Y., Hammer B., Iliadis L., Maglogiannis I. (eds) Artificial Neural Networks and Machine Learning – ICANN 2018. ICANN 2018. Lecture Notes in Computer Science, vol. 11141, pp. 280-288. Springer, Cham. DOI: https://doi.org/10.1007/978-3-030-01424-7_28
2017
- Morales G., Huamán S.G. and Telles J.: Cloud Detection for PERUSAT-1 Imagery Using Spectral and Texture Descriptors, ANN, and Panchromatic Fusion. In: Iano Y., Arthur R., Saotome O., Vieira Estrela V., Loschi H. (eds) Proceedings of the 3rd Brazilian Technology Symposium. BTSym 2017. Springer, Cham. DOI: https://doi.org/10.1007/978-3-319-93112-8_1
- Morales, G., Kemper, G. and Telles, J. (under review): Segmentation of Mauritia Flexuosa in aerial images using color and Gaussian Markov Random Field-based texture. Journal of Computing Science and Engineering, 2018.
- Ramírez, A., Valle, G.A., Romero, F., Jaimes, M.: Prediction of PVT Properties in Crude Oil Using Machine Learning Techniques MLT. In: SPE Latin America and Caribbean Petroleum Engineering Conference, Buenos Aires, Argentina, 2017.
2015
- Ramírez, A., Dianderas, E., Kemper, G.: Landscape units estimation in WorldView-2 images by using segmented urban areas, green areas and water bodies for monitoring variation/evolution of cities. In: 20th Symposium on Signal Processing, Images and Computer Vision, STSIVA 2015 – Conference Proceedings, Bogotá, 2015.
- Villafane, P., Telles, J., Diaz, D.: Desarrollo de un Método de Clasificación Automático de Elementos Urbanos y Segmentación en Zonas Agrícolas en Imágenes Satelitales WorldView-2. In: Proceedings of the 1st Brazilian Technology (BTSym), Campinas, 2015.
- Morales, G., Arteaga, D., Orduna, M., Kemper, G., Telles, J.: Un Algoritmo de Mejora de Imágenes Aéreas Adquiridas Vía UAV Orientado a Mejorar la Detección de Presencia de Aguajes Jóvenes en la Selva del Perú. In: Proceedings of the 1st Brazilian Technology (BTSym), Campinas, 2015.
2011
- Abanto, L.F., Kemper, G., Telles, J.: A New Method for Blocking Artifacts DetectionBased on Human Visual System Features. Inatel, 2011.
- Abanto, L.F., Kemper, G., Telles, J.: A novel fuzzy logic-based metric for audio quality assessment: Objective audio quality assessment. In: 2nd National Conference on Telecommunications (CONATEL), 2011.
Videos:
Presentación de Resultado de Proyectos
Tesis Itamar Salazar
Tesis Giorgio Morales
Tesis Daniel Arteaga
Proyecto Aguajales: Tic’s aplicadas a la conservación de la Biodiversidad
Proyecto MaiZ: Tic’s aplicadas a la Agricultura
Resumen Diciembre 2016
Proyecto Telemedicina
Proyecto Innóvate INICTEL-IIAP
Proyecto Innóvate INICTEL-INIA
TimberSpeciesPeru
The TimberSpeciesPeru dataset consists of 1575 images of fourteen timber species, which were acquired using two smartphones (Samsung Galaxy S6 and Motorola Moto X4) and a 60X Clip-On Microscope Magnifier Universal Lens. The fourteen species in this dataset are Machaerium inundatum (Aguano masha), Guazuma crinitia (Bolaina), Cariniana domestica (Cachimbo), Swietenia macrophylla (Caoba), Calycophyllum spruceanum (Capirona), Hura crepitans (Catahua amarilla), Cedrela odorata (Cedro), Cedrela fissilis (Cedro rojo), Peperomia inaequalifolia (Congona), Copaifera officinalis (Copaiba), Ceiba pentandra (Lupuna), Junglans neotropica (Nogal), Quararibea cordata (Sapote) and Cedrelinga cateniformes (Tornillo).
It can be downloaded from here:
Download TimberSpeciesPeru dataset
* To use this dataset, please cite the following paper:
M. P. E. Apolinario, D. A. Urcia Paredes and S. G. Huaman Bustamante, “Open Set Recognition of Timber Species Using Deep Learning for Embedded Systems,” in IEEE Latin America Transactions, vol. 17, no. 12, pp. 2005-2012, December 2019, doi: 10.1109/TLA.2019.9011545.
This TimberSpeciesPeru dataset is made available under a Creative Commons Attribution-NonCommercial-ShareAlike 4.0 International (CC BY-NC-SA 4.0) license.
CloudPeru
The CloudPeru dataset consists of 476,422 image patches labeled as ‘clouds’ or ‘non-clouds’, from which 207,963 are clouds and 268,459 non-clouds. These images were extracted from 15 different PERUSAT-1 scenes which were previously corrected to obtain surface reflectance products. Each image patch is size normalized to 27×27 pixels and has four channels: Red (0.63-0.7 μm), green (0.53-0.59 μm), blue (0.45-0.50 μm) and NIR (0.752-0.885 μm). The spatial resolution is 2.8 m per pixel. We split 95% of the data to create the training set, 2.5% to the validation set and 2.5% to the test set.
The dataset is encoded as an “.hdf5” file, which can be read from Matlab or from Python using the “h5py” library. It can be downloaded from here:
* To use this dataset, please cite the following paper:
Morales G., Huamán S.G., Telles J. (2018) Cloud Detection in High-Resolution Multispectral Satellite Imagery Using Deep Learning. In: Kůrková V., Manolopoulos Y., Hammer B., Iliadis L., Maglogiannis I. (eds) Artificial Neural Networks and Machine Learning – ICANN 2018. ICANN 2018. Lecture Notes in Computer Science, vol. 11141, pp. 280-288. Springer, Cham. DOI: https://doi.org/10.1007/978-3-030-01424-7_28
This CloudPeru dataset is made available under a Creative Commons Attribution-NonCommercial-ShareAlike 4.0 International (CC BY-NC-SA 4.0) license.
ShadowPeru
The ShadowPeru dataset consists of 400 image patches extracted from 20 different PERUSAT-1 scenes of variable area and from different geographies. Each image patch has a correspondent shadow mask, which was manually annotated. These scenes were were previously corrected to obtain surface reflectance products. The patches are size normalized to 256×256 pixels and have four channels: Red (0.63-0.7 μm), green (0.53-0.59 μm), blue (0.45-0.50 μm) and NIR (0.752-0.885 μm). The spatial resolution is 2.8 m per pixel. We split 90% of the data to create the training set, 5% to the validation set and 5% to the test set.
The dataset is encoded as an “.hdf5” file, which can be read from Matlab or from Python using the “h5py” library. It can be downloaded from here:
* To use this dataset, please cite the following paper:
Morales, G., Arteaga, D., Huamán, S., Telles, J. and Palomino, W.: Shadow Detection in High-Resolution Multispectral Satellite Imagery Using Generative Adversarial Networks. In: IEEE XXV International Conference on Electronics, Electrical Engineering and Computing (INTERCON), 2018. DOI: 10.1109/INTERCON.2018.8526416
This ShadowPeru dataset is made available under a Creative Commons Attribution-NonCommercial-ShareAlike 4.0 International (CC BY-NC-SA 4.0) license.
WoodSpeciesPeru
The WoodSpeciesPeru dataset consist of 46,000 image patches of seven wood species, which are labeled with indexes ranging from 0 to 6 that correspond to species Ceiba pentandra, Cariniana domestica, Swietenia macrophylla King, Ormosia coccinea, Aspidosperma macrocarpon, Dipteryx micrantha and Cedrelinga cateniformis, respectively. These images were extracted from 115 different samples of the transverse, radial and tangential sections of the wood acquired with a digital microscope CISNO.
Each image patch has a size of 128×128 pixels and a scale of 0.5mm per 100pixels. We split 70% of the data to create the training set, 15% for the validation set and 15% for the test set.
The dataset is encoded as an “.hdf5” file, wich can be read from Matlab or from Python using “h5py” library. It can be downloaded from here:
Download WoodSpeciesPeru dataset
* To use this dataset, please cite the following paper:
Apolinario M.P., Huamán S.G., Clostre G.: Deep Learning Applied to Identification of Commercial Timber Species from Peru. In: IEEE XXV International Conference on Electronics, Electrical Engineering and Computing (INTERCON), 2018. DOI: 10.1109/INTERCON.2018.8526457
This WoodSpeciesPeru dataset is made available under a Creative Commons Attribution-NonCommercial-ShareAlike 4.0 International (CC BY-NC-SA 4.0) license.
MauFlex Dataset
We acquired aerial images of Mauritia Flexuosa swamps (“aguajales”) south of the city of iquitos since 2015 to 2018. We selected the most 96 representative ones to create the dataset: 47 were acquired with a TurboAce UAV (Sony Nex-7 camera); 28, with a Mavic Pro UAV; and 21, with a SkyRanger UAV. Each image has a correspondent binary hand-drawn Mauritia Flexuosa mask that indicates with white color the presence of this palm. From these images, we extracted image patches of 512 x 512.
In order to analyze the images at different scales, we re-sized the images captured with the TurboAce UAV to 50% and 25% of the original size, due to the high level of details of these images. In addition, we used data augmentation to increase the dataset size in order to avoid overfitting problems; thus, we rotated each patch 90°, 180° and 270°. In this way, we created the MauFlex dataset, which is conformed by 25,248 image patches, each one with its correspondent binary mask. We split 95% of the data to create the training set, 2.5% to create the validation set and 2.5% to create the test set.
It can be downloaded from here:
This MauFlex dataset is made available under a Creative Commons Attribution-NonCommercial-ShareAlike 4.0 International (CC BY-NC-SA 4.0) license.
UNI-Crime Dataset
We constructed the UNI-Crime dataset based on the UCF-Crime dataset. For this, we discarded those multi-camera and repeated videos. Due to the fact that some videos are too long (three minutes or more) and others too short (20 seconds), we standardized the duration of our videos to 10 seconds. To do this, we trim each 10 useful seconds of the videos and classify them as robbery or non-robbery; by doing so, we can get multiple scenes of both classes from a single video, which strengthen our model, since it is less prone to overfitting. We also re-sized all the videos to 256 × 256 pixels and standardized the frame rate to 3 frames per second; that is, 30 frames per video, in order to avoid redundant information. In addition, we downloaded extra videos from Youtube, mainly from robberies or normal actions at stores. In the end, we collected 1421 videos: 1001 of non-robbery and 420 of robbery. A sample of frames from the dataset is shown
It can be downloaded from here:
This UNI-Crime dataset is made available under a Creative Commons Attribution-NonCommercial-ShareAlike 4.0 International (CC BY-NC-SA 4.0) license.
CloudPeru2
We used 153 PERUSAT-1 scenes of variable sizes (from 6176 x 6012 to 12722 x 9529 pixels) and from different geographies to extract 2800 image patches of 512 x 512 pixels and create the CloudPeru2 dataset. The scenes were previously orthorectificated and adjusted to reflectance values with atmosferic correction. Each image patch has a correspondent hand-drawn shadow mask. Nevertheless, for this work we used data augmentation to increase the dataset size in order to avoid overfitting problems. In that sense, we rotated each patch 90°, 180° and 270°, and flipped horizontally each one so that we get a total of 22,400 patches. We split 90% of the data to create the training set, 5% to the validation set and 5% to the test set.
It can be downloaded from here:
* To use this dataset, please cite the following paper:
Morales, G., Ramírez, A., and Telles, J. (submitted): End-to-end Cloud Segmentation in High-Resolution Multispectral Satellite Imagery Using Deep Learning. In: IEEE XXVI International Conference on Electronics, Electrical Engineering and Computing (INTERCON), 2019.
This CloudPeru2 dataset is made available under a Creative Commons Attribution-NonCommercial-ShareAlike 4.0 International (CC BY-NC-SA 4.0) license.
Derechos de Autor:
- Telles, J., Palomino, W., Brioso, U., Vargas, C., Morales, G., Ortega, I., Pacheco, A., Huamán, S.G. (2017). “Software de Identificación de Tres Patrones de Deforestación SI3PD”. LIMA, PERU. Registered at National Institute for the Defense of Competition and Protection of Intellectual Property (INDECOPI). Reg. Number: 01758-2017. File number: 003031-2017. Registration date: 13/11/2017.
- Morales, G., Bendayán, L., Sanjurjo, J., Castro, A., Kemper, G., Ponce, D., Telles, J. (2017). “Software de Teledetección de Palmeras de Aguajales”. LIMA, PERU. Registered at National Institute for the Defense of Competition and Protection of Intellectual Property (INDECOPI). Reg. Number: 00989-207. File number: 001120-2017. Registration date: 11/07/2017
- Diaz, D., Telles, J., Palomino, W., Injante, P. (2017). “Plataforma de Teledetección con Fines Agrícolas”. LIMA, PERU. Registered at National Institute for the Defense of Competition and Protection of Intellectual Property (INDECOPI). Reg. Number: 00156-207. File number: 001145-2017. Registration date: 25/01/2017